Bias in Space Domain Awareness - Knowing is Half the Battle!
It's easy for bias to creep into your Knowledge Management System. Especially in Space Domain Awareness. How can we identify and mitigate bias creep in our Space Domain Awareness Systems?
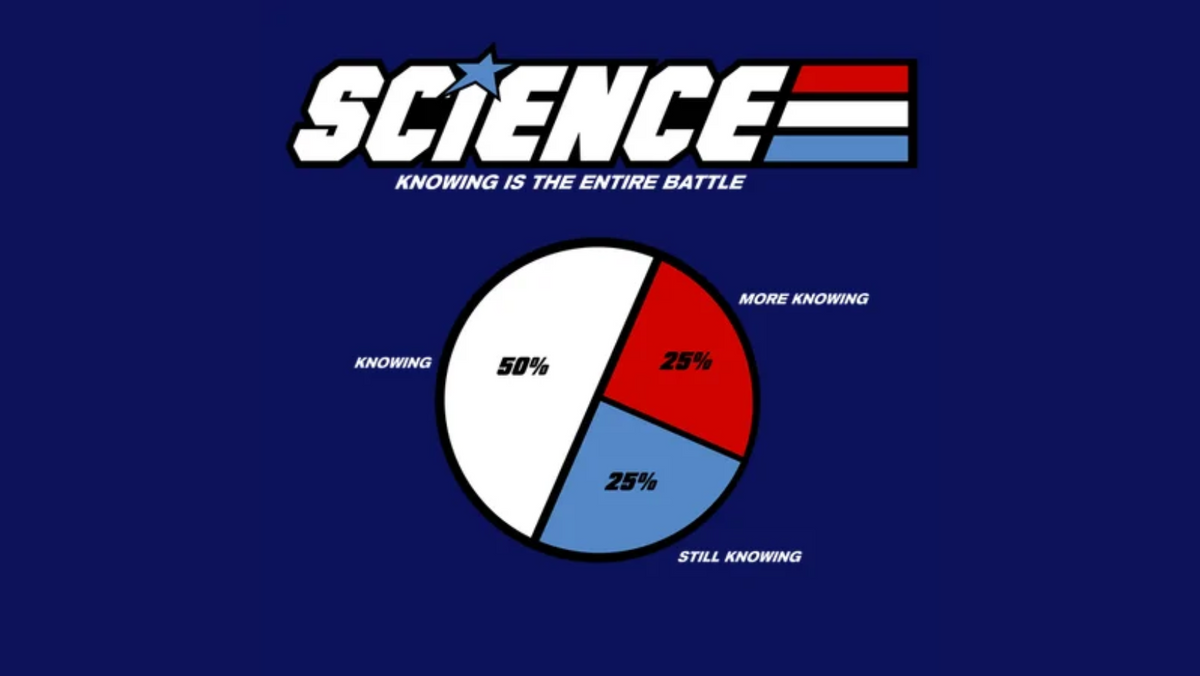
It's easy for bias to creep into your Knowledge Management System. Especially in Space Domain Awareness. Tasking sensors to obtain data is itself a decision - a decision where you use your existing knowledge to make data collection decisions. It's a loop in which biased knowledge tends to bias future knowledge production. How can we identify and mitigate bias creep in our Space Domain Awareness Systems?
Knowledge bias in Space Domain Awareness (SDA) Systems is a perennial problem. SDA sensor asset tasking is overwhelmed with possible questions to address and answer, making it vulnerable to decision-maker cognitive biases. Active steps in data collection and knowledge production must be taken to identify and mitigate SDA system and decision-maker bias.
To peel this onion, we need to examine 1) what bias is, 2) how it creeps into SDA systems and decision-making, and 3) what approaches to identify and mitigate bias we can use.
Let's do this.
1) What is Bias?
A bias is an incorrect perception of objective truth. The human mind and its many flaws leads to a long list of cognitive biases.
I'm not going to discuss all of these biases - for the purpose of this article I'll concentration on just a few of them:
Confirmation Bias
We want what we believe to be true. Choosing to focus on evidence that supports our beliefs is called Confirmation Bias.
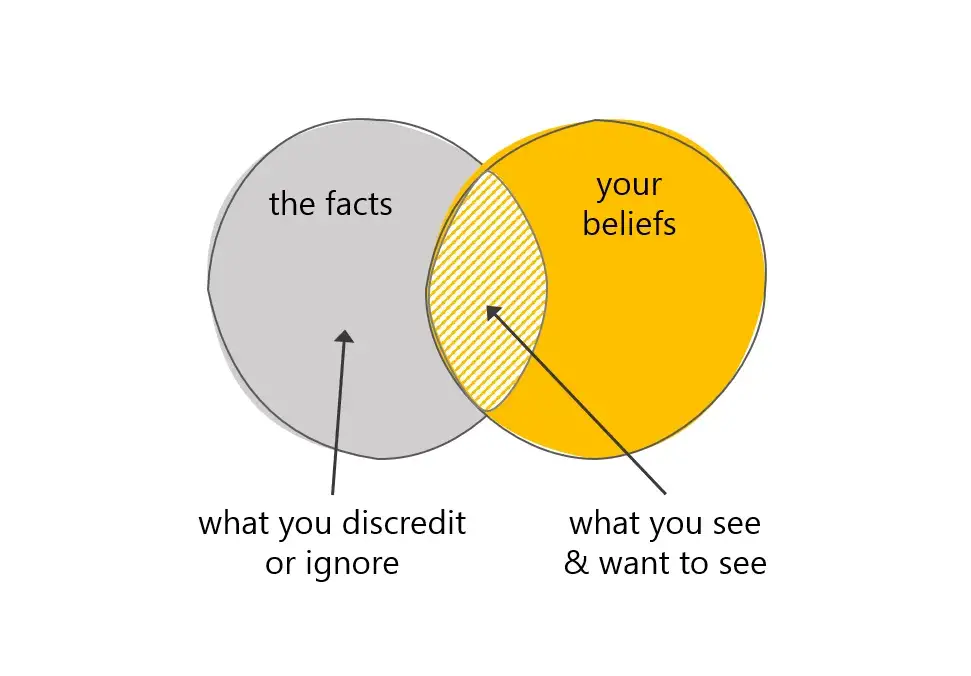
Availability Bias
It can be hard to resolve a question if you don't have any data. This particular problem leads to Availability Bias, wherein we tend to focus overmuch on hypotheses to which data exists or could be collected.
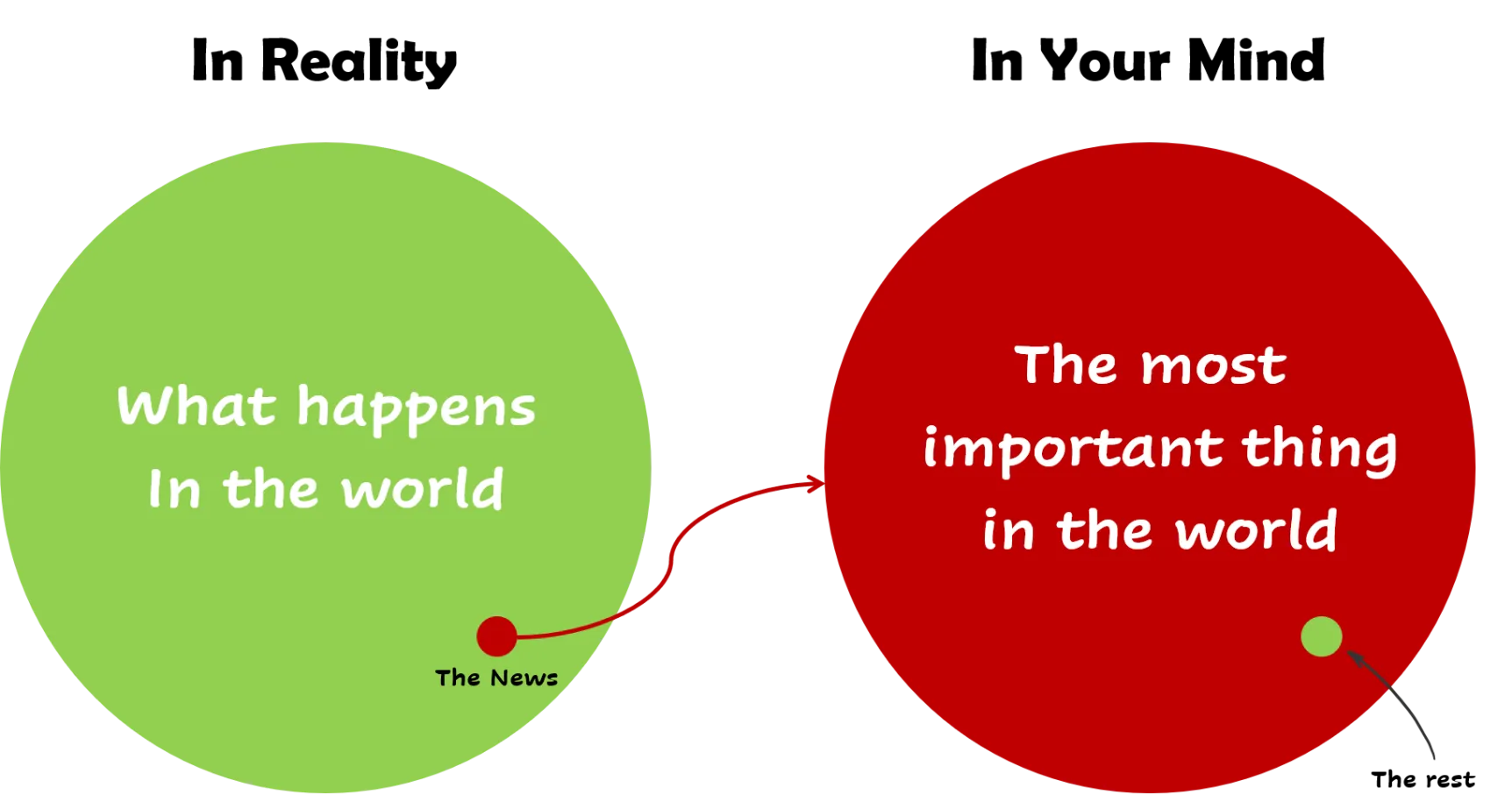
Availability bias is connected to Johari Windows and is related to unknown-knowns and unknown-unknowns.
Normalcy Bias
Sometimes we don't question why things are the way they are. Taking the status quo as given and natural is called a Normalcy Bias (related to Conservatism bias).
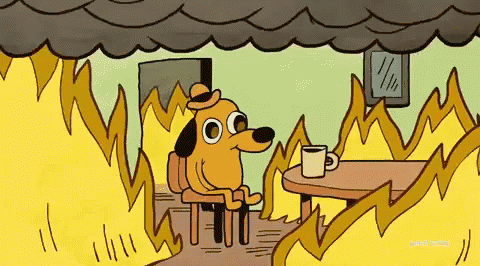
Yes, even if an SDA system is dysfunctional, it can be viewed as 'just fine' by its operators and dependent decision-makers.
Bias and Cognitive Dissonance in SDA Systems
Together, many of these biases can lead to cognitive dissonance and low-quality decision-making.
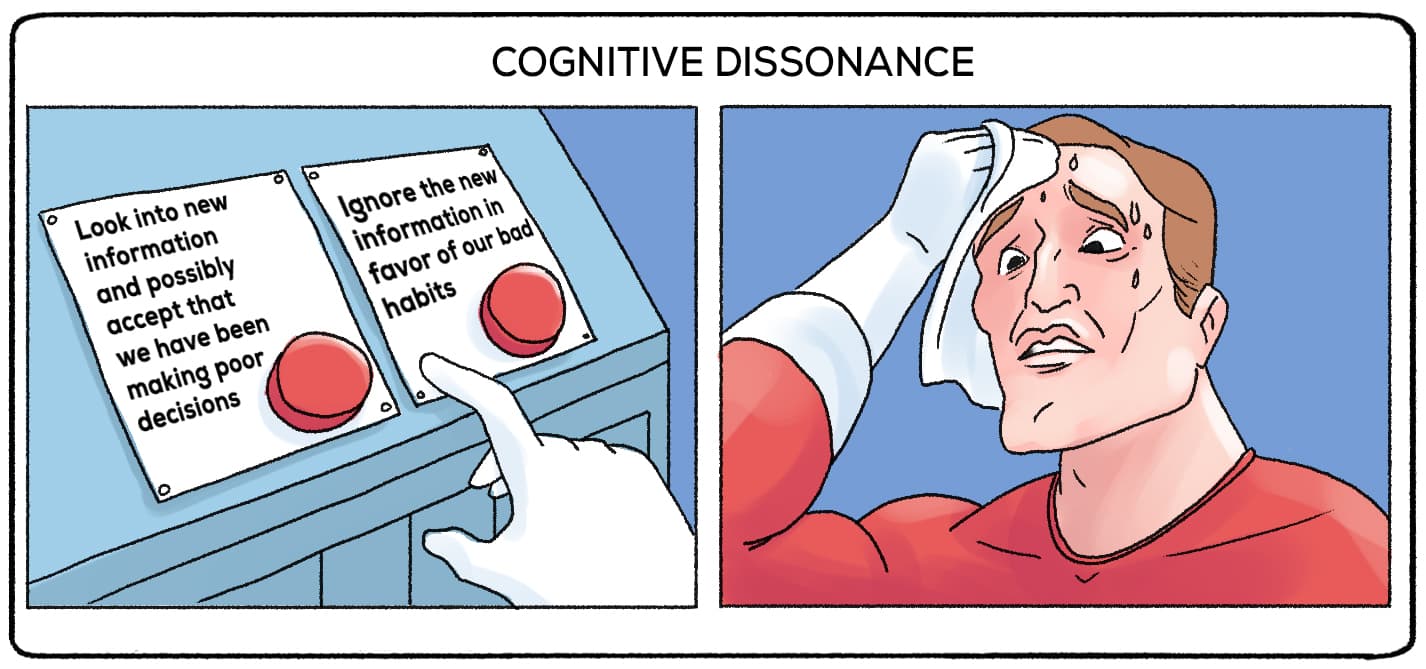
Hopefully, I don't need literary gymnastics to convince the reader that biases can lead to cognitive dissonance in SDA systems and resulting decision-making.
I wager that all of these biases are familiar with your own life experience. How do they affect SDA systems?
2) How Bias Creeps into our SDA Systems
There are infinite paths to corrupt SDA systems with bias. Or, at least as many ways as us humans can find ways to bias ourselves.
In this section I omit more traditional forms of bias induced by phenomenological effects (e.g., sensor bias). Sensor bias is well studied; I'd like to focus on human aspects.
There are a few specific bias pathways that bear discussion.
The Set of Infinite Questions Meets Finite Resources
If the number of possible tasks exceeds the capabilities of our sensing assets - a virtual certainty - we are on the horns of a two-pronged dilemma.
How do we form questions and choose the tasking for our systems?
Forming a Question is a Decision
The biggest problem here is that we can only interrogate questions we can conceive of. This is a prime avenue for Availability Bias and Normalcy Bias. This relates directly to the level of convergent and divergent thinking occurring in the SDA system. This question of known-knowns, known-unknowns, unknown-unknowns, and unknown-knowns (e.g., the Johari Window) is tied directly to divergent thinking in hypothesis generation.
We don't look for events, threats, or hazards that we haven't thought of - that are not part of our explicit or implicit knowledge base.
"This is the way we've always done it" is the herald of bias, and makes SDA systems vulnerable to failures of imagination - those failures and errors of omission that no one saw coming.
Choosing not to ask new questions is in itself a decision - in particular, an error of omission (read more about omission bias).
"One potential reason behind Omission Bias is that we often do not know about the losses that arise from Errors of Omission." (pg. 111, [[Maxims on Thinking Analytically]])
Errors of Omission in question ideation directly contribute to SDA system bias, but in the most insidious of means - we haven't thought of them.
Tasking Assets is Another Decision
Once we've decided to ask what we hope to be a good question, we are faced with another bias-inducing problem: how to we task our assets to resolve the question?
Confirmation Bias is our biggest nemesis when tasking sensors to obtain data and produce knowledge. If we only look for data confirming or disconfirming a hypothesis we risk arriving at an incomplete or incorrect conclusion.
Further, it is often the case that data to disconfirm or confirm a hypothesis is incomplete or cannot be collected, leading to Availability Bias. Does a missed detection mean that the object maneuvered, isn't reflecting as many photons, or something else (none of the above)?
Now that we've possibly lost a measure of faith in our own SDA systems, let's look at how to address these problems.
3) Identifying and Mitigating Bias in SDA Systems
Systematized Bias Mitigation
An irony in life is that being aware of your biases doesn't always allow you to eliminate or mitigate them. This so-called G.I. Joe Bias (because knowing is half the battle) is an excellent topic for a future article.
For now, let's focus on systems to mitigate the biases we've identified in terms of decision-making in question ideation and asset tasking.
Gaming Against Normalcy and Availability Bias
Question ideation - central to reducing the set of unknown-unknowns and unknown-knowns - is challenging to do well.
One methodology that has been successfully used in the past has been professional wargaming. In professional wargames, scenarios are constructed to interrogate specific questions, such as those pertaining to tactics, operations, or grand strategy. In the course of running these 'exercises,' professional wargaming can induce 'out of the box' or divergent thinking in the players.
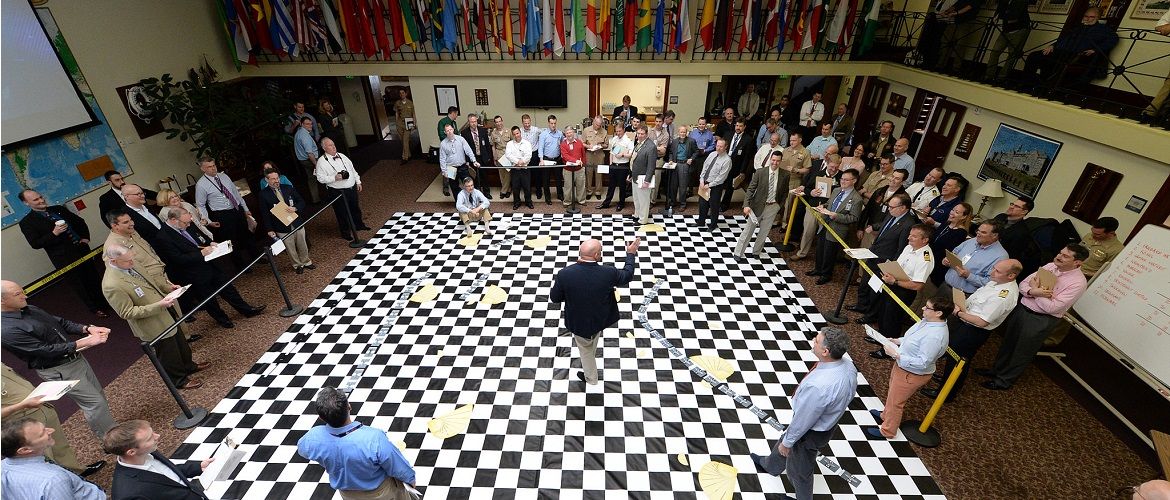
New questions can be posed in-situ by the players. Players and adjudicators conduct hot washes and analyses to identify lessons learned and new questions. Creative and divergent thinking is encouraged, recorded, and harnessed.
A famous anecdote from United States Navy Admiral Chester W. Nimitz years after the second world war attests to the utility of professional wargames in the 1920s and 1930s in mitigating unknown-unknowns and unknown-knowns:
“The war with Japan had been re-enacted in the game rooms here by so many people and in so many different ways, [...] that nothing that happened during the war was a surprise—absolutely nothing except the kamikaze tactics towards the end of the war; we had not visualized those." Admiral Chester W. Nimitz, 1960
SDA system operators and decision-makers: sharpen your pencils and participate in gaming exercises regularly.
Laying the Law Down on Confirmation Bias
Suppose we have a SDA question in hand that we'd like to resolve. How can we trust ourselves to task assets to resolve it without our own beliefs and biases insinuating themselves?
Can we trust ourselves to remain unattached to the outcome of a particular question? Are we secretly hoping that a hypothesis will be true or false, for whatever reason?
This is not a new problem to the human condition. It's as old as agriculture and famously addressed by Hammurabi, King of Babylon in 1750 BC.
Since antiquity humans have labored to remove bias from hypothesis resolution. I'm talking about judicial systems. In the United States, we call this the Federal Judiciary, and it's so important that we've made it a branch of government.
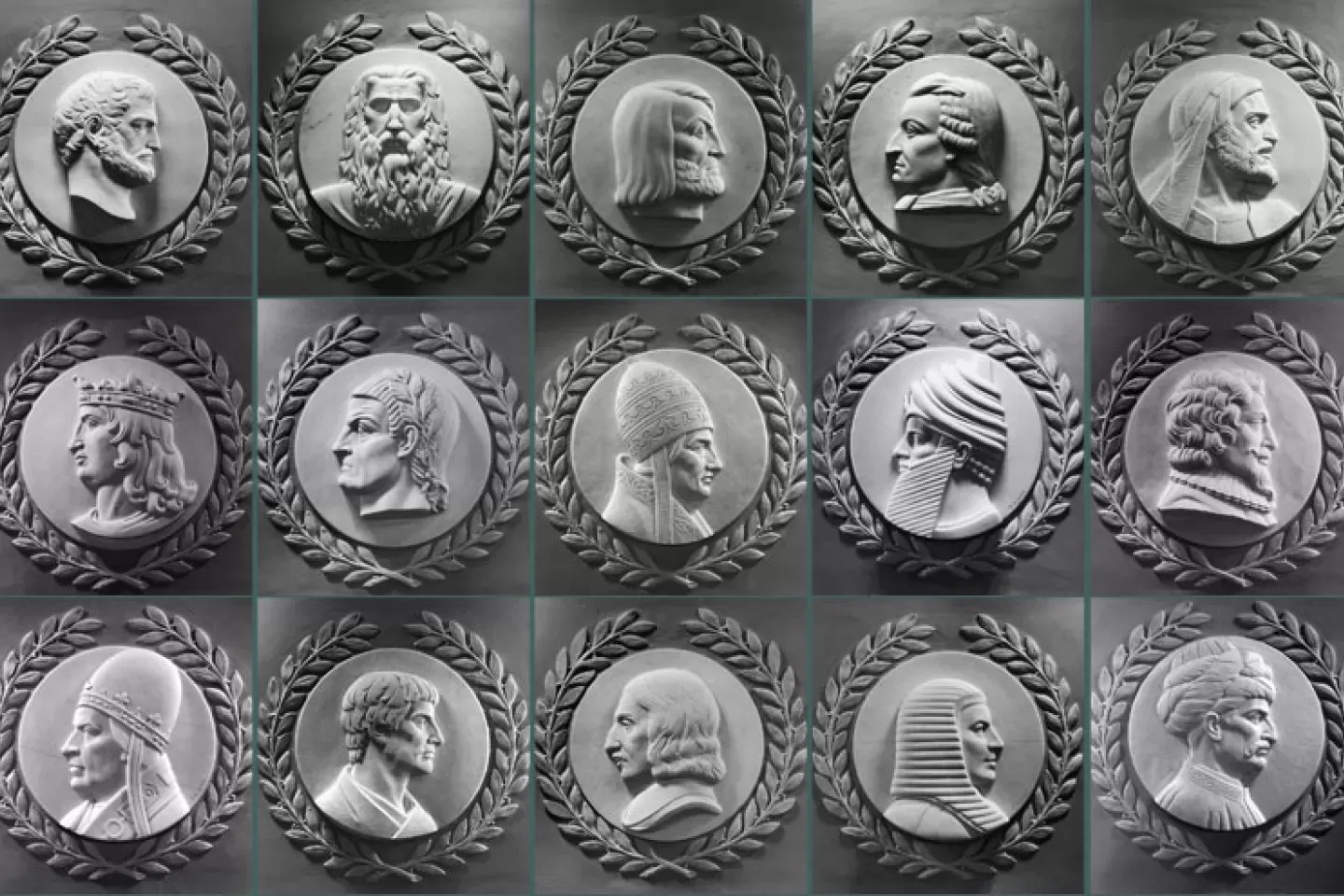
At my own peril, I would describe the judicial approach of mitigating bias as 1) simplifying the question at hand to its most essential nature - a binary 'true / false' outcome, and 2) assigning agents to simultaneously prove the hypothesis as true or false.
A question could be 'the defendant is guilty of an accused crime.' The two agents then argue that the hypothesis is false (defense) or true (prosecution).
Wait, so now we need a courtroom in our SDA operations center? No, not quite.
For simple hypotheses / questions, previous research has operationally shown how to task sensors to resolve them using Judicial Evidential Reasoning (JER). At present, JER is complex, computationally intensive, and depends upon experienced scientists and engineers generating belief mass functions (BMFs) to map sensor information to specific hypotheses. This bespoke process is labor intensive.
In research, JER has been used to resolve maneuver malfunctions in orbit insertion and Raven-Class telescope tasking for space object custody and maneuver anomaly detection.
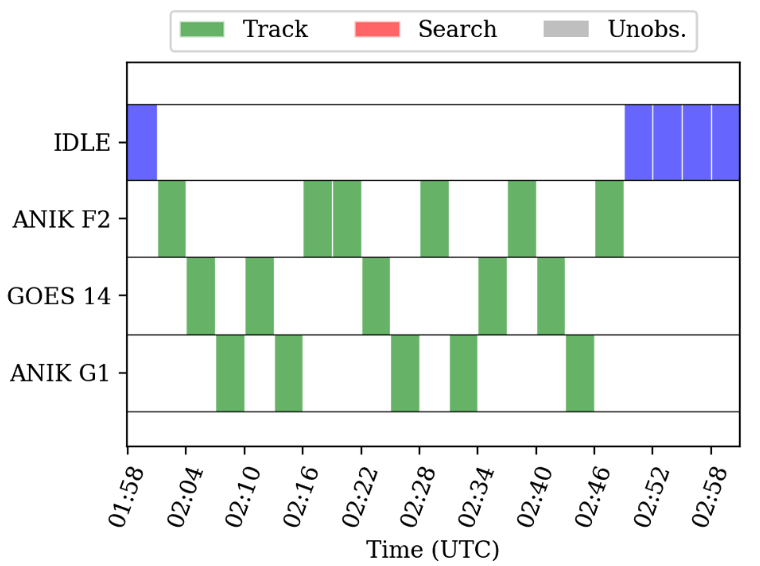
When considering complex hypotheses, I suggest decomposing them using logic tools and formal methods. This can generate individually testable true / false hypotheses.
A much simpler and possible more accessible approach is to assign operators as proponents of hypothesis truthness / falseness, and let them present their cases to an adjudicator.
If a hypothesis cannot be tested, that suggests either a new sensing asset / modality is needed, or a surrogate hypothesis must be substituted (beware surrogate hypotheses - there lies another form of bias for the unwary!).
This is an area for future research, but as G.I. Joe (and the aptly named G.I. Joe Bias) says, when it comes to biases "knowing is half the battle!"
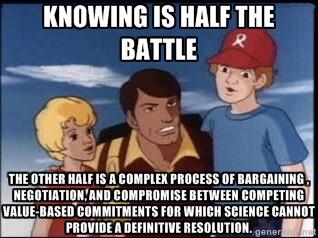
Let's wrap this up into a digestible package.
Summary
From this brief discussion of bias in Space Domain Awareness (SDA) systems, we can form three principal observations:
- There are many ways for humans and our SDA systems to misrepresent, misunderstand, and misjudge objective reality in the space domain. These biases are unavoidable, but can be mitigated.
- SDA systems suffer from bias in generating questions to resolve. This can be addressed by professional wargaming.
- Sensor tasking can result in bias because we must choose what data to collect and what uncollected data to ignore. We can borrow from the judiciary to use Judicial Evidential Reasoning, mitigating finite sensing asset constraints in SDA systems.
These articles are always longer than I expect, though hopefully you've enjoyed reading!
Subscribe to the Newsletter
If you enjoy this content, show your support by subscribing to the free weekly newsletter, which includes the weekly articles as well as additional comments from me. There are great reasons to do so, and subscriptions give me motivation to continue writing these articles! Subscribe today!